AI Text Classifier
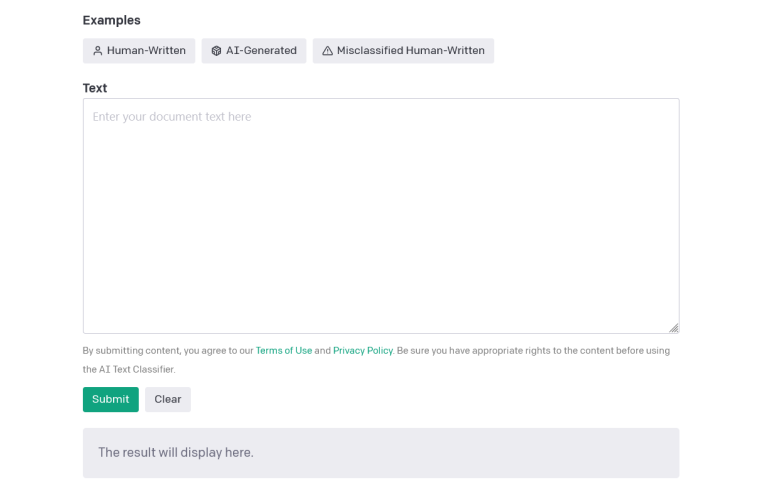
Category
Protect Your Online Reputation: Ensure Authenticity with AI Text Classification
Pricing
Pros
- Efficiency: Automate time-consuming manual tasks, saving time and resources.
- Accuracy: Often outperform humans in classifying large text volumes quickly and consistently.
- Scalability: Handle vast amounts of data efficiently, ideal for big data applications.
- Insights: Uncover hidden patterns and trends that might be missed by manual analysis.
- Adaptability: Can be customized to specific needs and domains through model training.
- Continuous Improvement: Learn from new data and potentially improve accuracy over time.
Cons
- Bias: Risk of inheriting biases from training data, leading to unfair or discriminatory outcomes.
- Transparency: Limited visibility into decision-making processes, hindering explainability and trust.
- Overreliance: Overdependence on AI can lead to complacency and reduced human oversight.
- Data Quality: Reliant on high-quality, unbiased training data for accurate results.
- Interpretability: Understanding why a model makes certain classifications can be challenging, limiting trust and adoption.
Related Tools 🛠️
SEOLligence appears to be a comprehensive SEO (Search Engine
The corporate world is continuously evolving, driven by advancements
SEO Writing AI offers a compelling solution for content
Keyword Surfer is a browser extension (likely for Chrome)
Quick Creator presents a promising tool for streamlining content
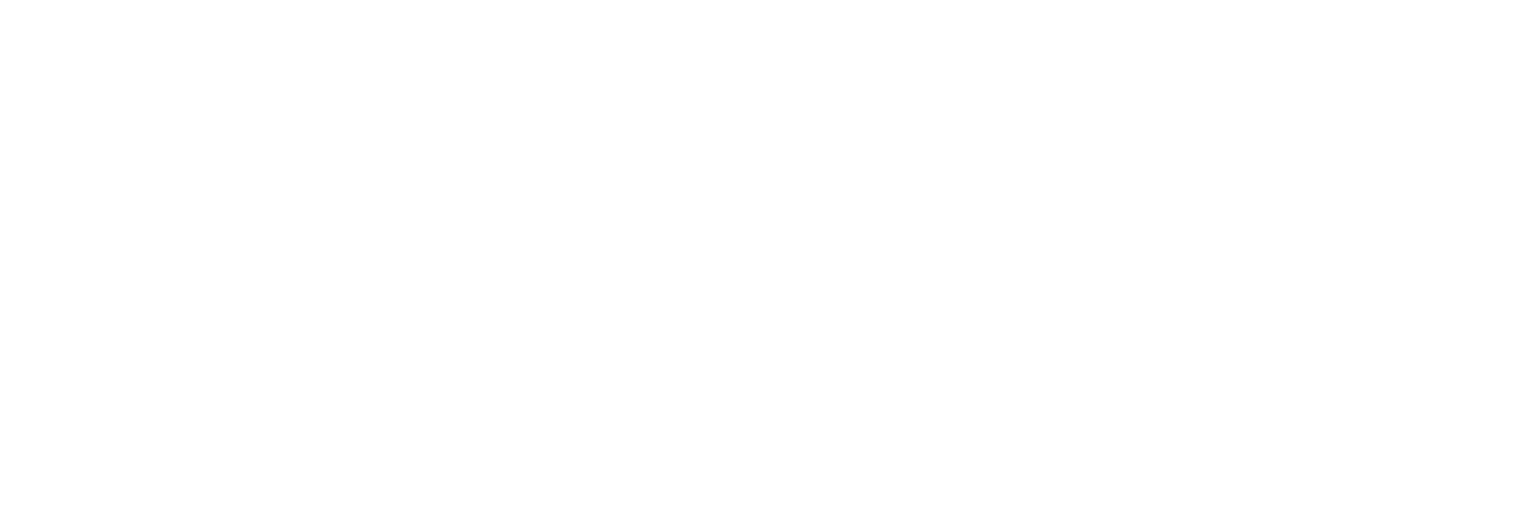
Your trust fuels our growth! When you shop through our affiliate links, it helps us keep creating quality content, at no additional cost to you. Thanks for reading!
© All Rights Reserved 2024